Only 38% of rail customers in the UK are happy with how rail firms handle delays that are frequently brought on by equipment breakdowns. It aptly illustrates how, despite maintenance making up a large portion of the train industry’s costs, the current methods cannot deliver a sufficient level of service. Predictive maintenance has emerged as the most successful and promising maintenance technique in the railway industry with the emergence of the internet of things (IoT). However, the majority of railway equipment worldwide still lacks even real-time monitoring capabilities. According to Julia Seredovich, business operations manager of Professional Software Associates Inc., how serious is this situation?
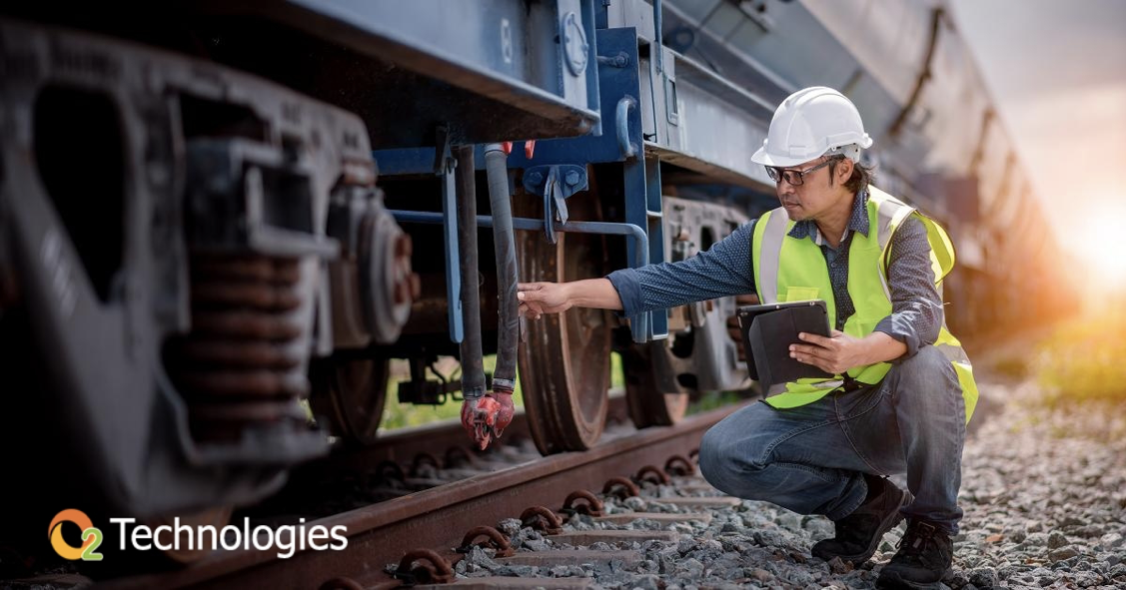
Depending on the volume of traffic on a specific railway network, different regions experience the need for Predictive Maintenance (PdM) in different ways. The infrastructure must be in tip-top shape to handle such an increase in loads in the US, where there has been a huge growth in demand for rail transportation over the previous five years. If you wish to minimize unexpected failures and, ideally, eliminate them, predictive maintenance is a practice that is difficult to avoid in such circumstances. Can we make this happen? In order to find out, PSA provides its knowledge on PdM use cases and IoT technologies that have shown to be the most effective PdM solutions for rail, concentrating on how to approach them sensibly.
Predictive maintenance’s use in railroads
- Predictive maintenance is a strategy that permits service work to be done solely on vulnerable parts of structures or equipment before the likelihood of their breakdown falls below a maintainable level. Unlike scheduled maintenance based on statistics, this method is based on the processing of actual, real-time data of individual equipment. The basic idea behind PdM is that by calculating the machine’s remaining useful life (RUL) and scheduling maintenance appropriately, we can monitor changes in the machine’s characteristics in real-time.
The firm needs specialized research and development activities in order to make PdM cost-effective even though the data required for its execution can be entirely delivered automatically through IoT. Technically, the following elements should be included in solutions for predictive maintenance in railways:
- Exterior and sensor-based data gathering. Rail assets can be remotely and continuously monitored by adding IoT sensors to them. The more data we collect, the more opportunities we have to develop a strong analytical model that can make the most precise forecasts. Additionally, the accuracy improves when we consider more variables by including diverse data from multiple sources. Building a practical predictive model may require historical data from field inspections, engineering data on the mechanical parameters, data from remote control systems, data from asset management, and external data like weather or GPS coordinates.
- Transmitting data to the cloud. To transfer the data created in the field in real-time or almost real-time, reliable connectivity should be established. It may be necessary to install additional hardware, such as a radio tower, implement cellular-based data transmission, and use cable or wireless connection for this; these are important considerations. For PdM, machine learning is typically implemented using MQTT, XMPP, AMQP, or WebSocket, whilst train-to-ground applications can be created utilizing LTE or WLAN.
- Examination of data using AI. You can create a strong predictive-analytical model and then deploy it in the cloud by identifying the pertinent status indicators that signal impending failure. As a result, real-time field data may be collected and analyzed in a cloud application to generate reports and make precise forecasts about RUL.
Positive outcomes are already evident, despite the fact that predictive maintenance in rail has only recently begun to acquire steam. Additionally, by using the best practices available, the operator can maximize the benefits of PdM implementation while minimizing iterations and trial-and-error processes.
Use cases for predictive maintenance in railroads
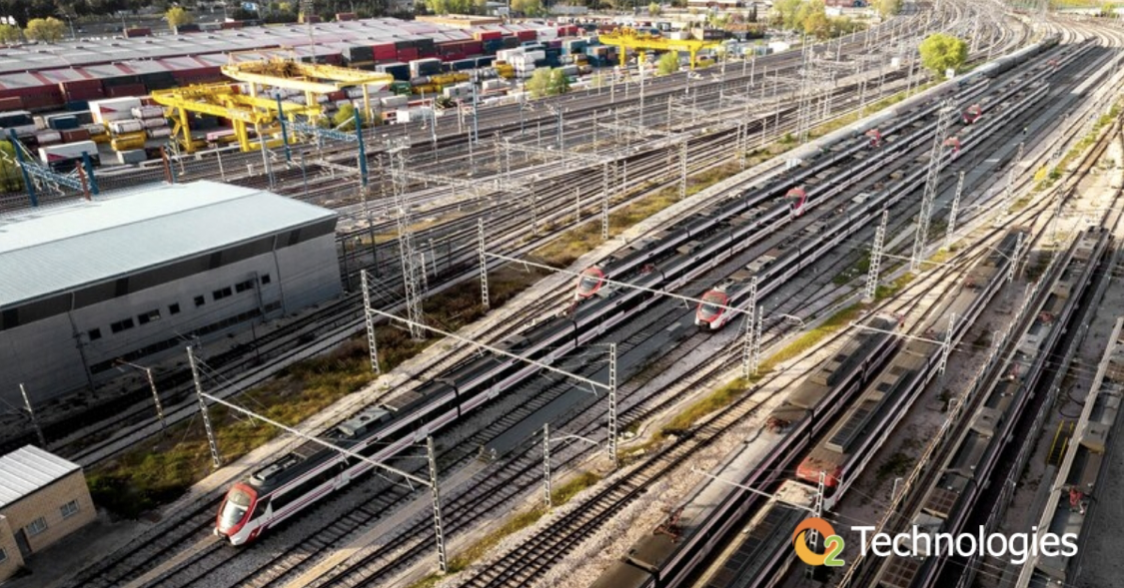
Predictive Maintenance in Railways includes engineering structures as well as digital and mechanical equipment. These parts are prime candidates for a Predictive Maintenance installation because they can be regarded as both mission- and safety-critical. Every scenario requires a slightly different strategy, but the basic idea remains the same. We assess the electronic health, functional health, and structural integrity of digital gadgets as well as their mechanical and functional health. Since non-destructive inspections may be performed on trains without causing damage to the structure or its parts, predictive maintenance is advised for this industry as well.
Maintenance planning for level crossings
Failures at level crossings top the list of factors that lead to rail accidents in Europe. Due to a lack of digitalization and oversight, the public can only learn about a crossing breakdown after reporting. Even real-time monitoring can dramatically shorten the time it takes to respond to an issue, but PdM opens up more complex possibilities. For instance, by installing gear at crossings that detects the gate opening angle, it is possible to monitor the angle’s decline over time and correlate it with impending failure. Given that level crossings deteriorate progressively, the algorithm assumes that there is still useful life left. It is possible to plan maintenance tasks automatically.
RUL for the gate control device can also be determined by observing current spikes, erratic voltage, high hardware temperatures, and component oxidation. PdM technologies can monitor how an item’s performance deteriorates and produce a precise prediction about when it will break down.
Bridge maintenance that is preventative
The most hazardous, expensive, and difficult to fix failure is when a railway bridge’s structural integrity is violated. Sadly, problems with the structural integrity of rail bridges are unavoidable since actual loads typically exceed design loads and operating conditions are frequently difficult. To produce precise predictions about when a bridge would break, IoT-based analytical tools can combine data on static and dynamic loads, the quality of materials, and the actual condition of the bridge. The model can be expanded with additional characteristics, such as bridge behavior during seismic events, rail profile anomalies, etc., after the bridge reactions to various loads have been simulated.
Major flaws that could lead to a bridge collapse do not develop overnight. They are the result of tiny cracks that have been compromising the bridge’s structural integrity for a few weeks or months. Vibration diagnostics and ongoing visual tracking of displacement, deviation, and tilt have been shown to be effective in this regard.
Tunnels’ predictive maintenance
Rail tunnels may experience drainage issues, seepage, cracks, concrete delamination, corrosion of the steel, delamination of the tunnel lining, and structural failure. In particular, corrosion of the steel reinforcement is frequently brought on by water leaks in the tunnel’s concrete lining. Ultrasonic sensors and radars assess speed in the material to monitor its structural integrity. Magnetic and electrical examinations quickly identify corrosion. IoT sensors can give visual and sound inspections to identify stratification of the concrete. You can acquire a comprehensive understanding of tunnel behavior over time and optimize maintenance plans by adding information on maintenance and repair history, external loads, source of failure, and so on.
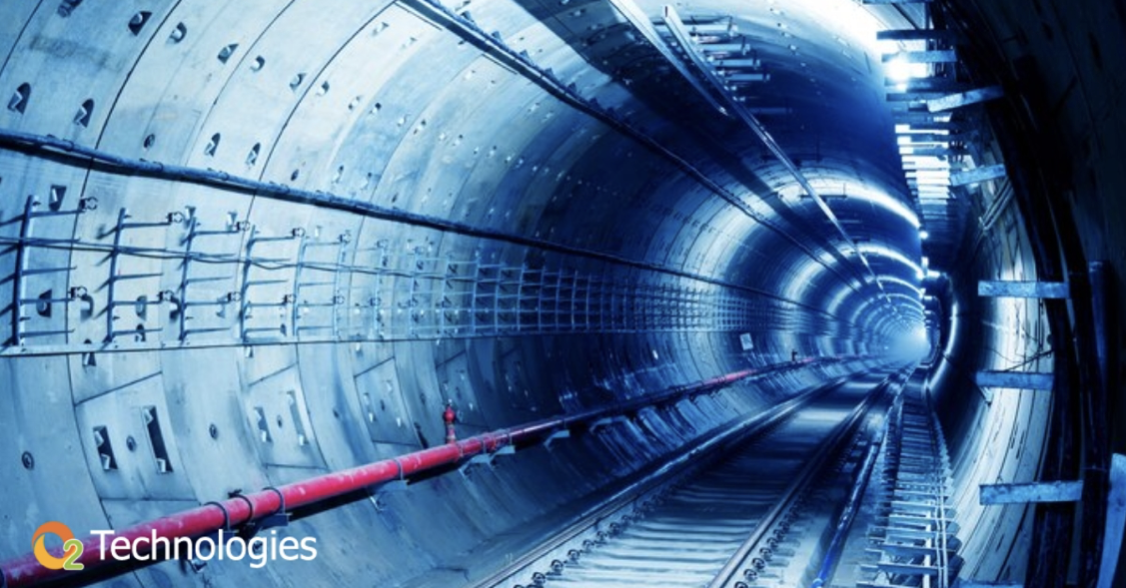
Maintenance planning for signalling infrastructure
We first discuss failures resulting from external variables, such as weather conditions, for track circuits, railroad switches, and axle counters. Therefore, the focus of their real-time monitoring is on safeguarding against contamination that could cause ice, corrosion, etc. Given that it is difficult to predict weather conditions in advance, we are actually talking more about condition-based maintenance than predictive maintenance here. An IoT-based management platform can quickly provide the alarm on it, warning dispatchers and maintenance teams of difficulties, by identifying any type of debris as soon as it emerges.
It is beneficial to outfit railway signalling solutions with additional sensors so that maintenance requirements may be fully understood. As a result, all the crucial information, including voltages in track circuits, current power usage, point shifting time, and cable resistance, will swiftly arrive at the control center, enabling accurate monitoring. “Promptly” is the operative term here because it minimizes delays-related costs and drastically cuts incident response time even in the event that a breakdown still occurs.
IoT has a practical method for bringing ancient systems online that makes use of edge devices that support both antiquated and contemporary communication protocols.
Rail industry’s predictive maintenance: A summary
- Although it has already shown its utility for several rail components, predictive maintenance has only just begun to gain traction for rail applications.
- When implementing PdM for rail assets, it’s essential to provide the data as completely as possible, including engineering, maintenance, external, and control data at the very least; to ensure stable connectivity, consider harsh exploitation conditions; and to give yourself enough time to build a practical analytical model for each type of asset.
- You may obtain continuous monitoring of the structural health of engineering structures by using predictive maintenance, which can be applied to them. This structural health can be determined using visual, vibrational, acoustic, magnetic, and other inspections. This makes it possible to detect small inclinations, deflections, or fissures before they become serious problems.
- PdM for control devices enables tracking of performance alterations that could result in failure. For more details about IOT services talk to our experts today!